Papers
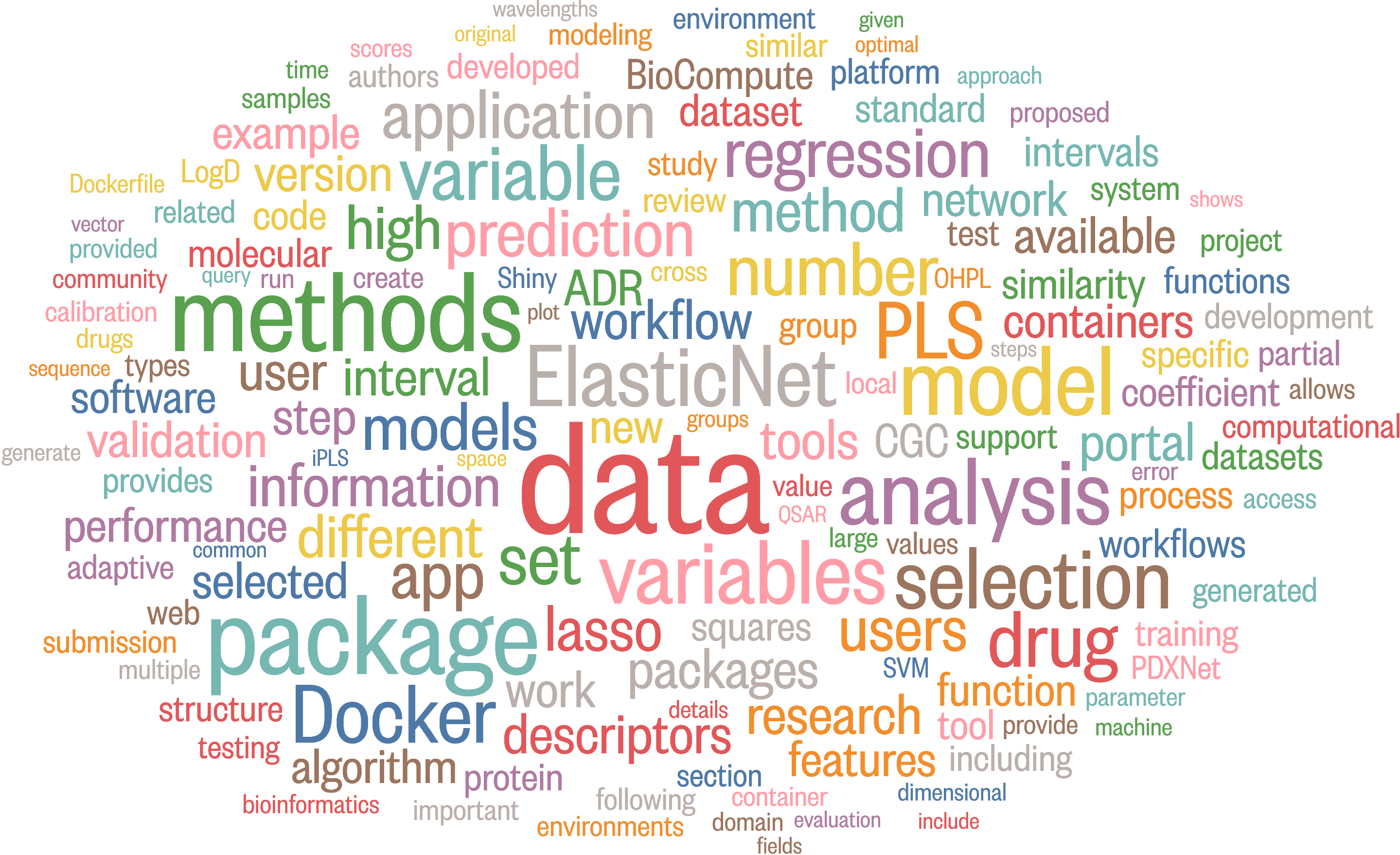
Word cloud visualization of the keywords in my publications.
Google Scholar | ORCID | ResearchGate | Download BibTeX file
2024 | 2023 | 2022 | 2021 | 2020 | 2019 | 2018 | 2017 | 2016 | 2015
2024
- Some Group Sequential Trials From Industry Over the Last 30 Years
Keaven M. Anderson, Yujie Zhao, Nan Xiao, Joy Ge, Harlan F. Weisman
Statistics in Biopharmaceutical Research 16(3): 281–293, 2024
PDF (Accepted Manuscript)
2023
- Electronic Common Technical Document Submission With Analysis Using R
Yujie Zhao, Nan Xiao, Keaven Anderson, Yilong Zhang
Clinical Trials 20(1): 89–92, 2023 - Building an Internal R Package for Statistical Analysis and Reporting in Clinical Trials: A SAS User’s Perspective
Huei-Ling Chen, Heng Zhou, Nan Xiao
PharmaSUG 2023 (Best Paper Award)
2022
- A Multilingual Shiny App for Drug Labelling in Worldwide Submission
Jinchun Zhang, Aiming Yang, Yiwen Luo, Nan Xiao, Yilong Zhang
PharmaSUG 2022 - PDXNet Portal: Patient-Derived Xenograft Model, Data, Workflow and Tool Discovery
Soner Koc, Michael W Lloyd, Jeffrey W Grover, Nan Xiao, Sara Seepo, Sai Lakshmi Subramanian, Manisha Ray, Christian Frech, John DiGiovanna, Phillip Webster, Steven Neuhauser, Anuj Srivastava, Xing Yi Woo, Brian J Sanderson, Brian White, Paul Lott, Lacey E Dobrolecki, Heidi Dowst, PDXNet Consortium, Yvonne A Evrard, Tiffany A Wallace, Jeffrey A Moscow, James H Doroshow, Nicholas Mitsiades, Salma Kaochar, Chong-xian Pan, Moon S Chen, Luis Carvajal-Carmona, Alana L Welm, Bryan E Welm, Michael T Lewis, Ramaswamy Govindan, Li Ding, Shunqiang Li, Meenhard Herlyn, Michael A Davies, Jack Roth, Funda Meric-Bernstam, Peter N Robinson, Carol J Bult, Brandi Davis-Dusenbery, Dennis A Dean, II, Jeffrey H Chuang
NAR Cancer 4(2), 2022
2021
- Bioinformatics Tools Developed To Support BioCompute Objects
Janisha A Patel, Dennis A Dean, Charles Hadley King, Nan Xiao, Soner Koc, Ekaterina Minina, Anton Golikov, Phillip Brooks, Robel Kahsay, Rahi Navelkar, Manisha Ray, Dave Roberson, Chris Armstrong, Raja Mazumder, Jonathon Keeney
Database 2021: 1–13, 2021
2020
- BCO App: Tools for Generating BioCompute Objects From Next-Generation Sequencing Workflows and Computations [version 1; peer review: 2 approved]
Nan Xiao, Soner Koc, David Roberson, Phillip Brooks, Manisha Ray, Dennis Dean
F1000Research, 9:1144, 2020 - The Rockerverse: Packages and Applications for Containerisation With R
Daniel Nüst, Dirk Eddelbuettel, Dom Bennett, Robrecht Cannoodt, Dav Clark, Gergely Daróczi, Mark Edmondson, Colin Fay, Ellis Hughes, Lars Kjeldgaard, Sean Lopp, Ben Marwick, Heather Nolis, Jacqueline Nolis, Hong Ooi, Karthik Ram, Noam Ross, Lori Shepherd, Péter Sólymos, Tyson Lee Swetnam, Nitesh Turaga, Charlotte Van Petegem, Jason Williams, Craig Willis, Nan Xiao
The R Journal 12(1): 437–461, 2020 - Strengthening the BioCompute Standard by Crowdsourcing on PrecisionFDA
Sarah H Stephens, Charles Hadley King IV, Sean Watford, Janisha Patel, Dennis A. Dean II, Soner Koc, Nan Xiao, Eric F Donaldson, Elaine E Thompson, Anjan Purkayastha, Raja Mazumder, Elaine Johanson, Jonathon Keeney
bioRxiv 2020.11.02.365528
2019
- Collaboration Patterns and Network in Chemometrics
Chuan-Quan Li, Nan Xiao, Ye Wen, Shi-Hui He, Yuan‐Da Xu, You-Wu Lin, Hong-Dong Li, Qing-Song Xu
Chemometrics and Intelligent Laboratory Systems 191: 21–29, 2019
PDF
2018
- dml: Distance Metric Learning in R
Yuan Tang, Tao Gao, Nan Xiao
Journal of Open Source Software 3(30): 1036, 2018 - A Selective Review and Comparison for Interval Variable Selection in Spectroscopic Modeling
Li-Li Wang, You-Wu Lin, Xu-Fei Wang, Nan Xiao, Yuan-Da Xu, Hong-Dong Li, Qing-Song Xu
Chemometrics and Intelligent Laboratory Systems 172: 229–240, 2018
PDF
2017
- Ordered Homogeneity Pursuit Lasso for Group Variable Selection With Applications to Spectroscopic Data
You-Wu Lin, Nan Xiao, Li-Li Wang, Chuan-Quan Li, Qing-Song Xu
Chemometrics and Intelligent Laboratory Systems 168: 62–71, 2017
PDF | Website | R package
2016
- A Novel Local Manifold-Ranking Based k-NN for Modeling the Regression Between Bioactivity and Molecular Descriptors
Liang Shen, Dong-Sheng Cao, Qing-Song Xu, Xin Huang, Nan Xiao, Yi-Zeng Liang
Chemometrics and Intelligent Laboratory Systems 151: 71–77, 2016
PDF - hdnom: Building Nomograms for Penalized Cox Models With High-Dimensional Survival Data
Nan Xiao, Qing-Song Xu, Miao-Zhu Li
bioRxiv 065524
2015
- Multi-Step Adaptive Elastic-Net: Reducing False Positives in High-Dimensional Variable Selection
Nan Xiao, Qing-Song Xu
Journal of Statistical Computation and Simulation 85(18): 3755–3765, 2015
PDF | R package - protr/ProtrWeb: R Package and Web Server for Generating Various Numerical Representation Schemes of Protein Sequence
Nan Xiao, Dong-Sheng Cao, Min-Feng Zhu, Qing-Song Xu
Bioinformatics 31(11): 1857–1859, 2015
PDF | R package | Shiny app - Rcpi: R/Bioconductor Package To Generate Various Descriptors of Proteins, Compounds and Their Interactions
Dong-Sheng Cao, Nan Xiao, Qing-Song Xu, Alex F. Chen
Bioinformatics 31(2): 279–281, 2015
PDF | R package - Integrating Multiple Evidence Sources To Predict Adverse Drug Reactions Based on Systems Pharmacology Model
Dong-Sheng Cao, Nan Xiao, Yuan-Jian Li, Wen-Bin Zeng, Yi-Zeng Liang, Ai-Ping Lu, Qing-Song Xu, Alex F. Chen
CPT: Pharmacometrics & Systems Pharmacology 4(9): 498–506, 2015
PDF | Code and dataset - In Silico Evaluation of logD7.4 and Comparison With Other Prediction Methods
Jian-Bing Wang, Dong-Sheng Cao, Min-Feng Zhu, Yong-Huan Yun, Nan Xiao, Yi-Zeng Liang
Journal of Chemometrics 29(7): 389–398, 2015
PDF | Dataset