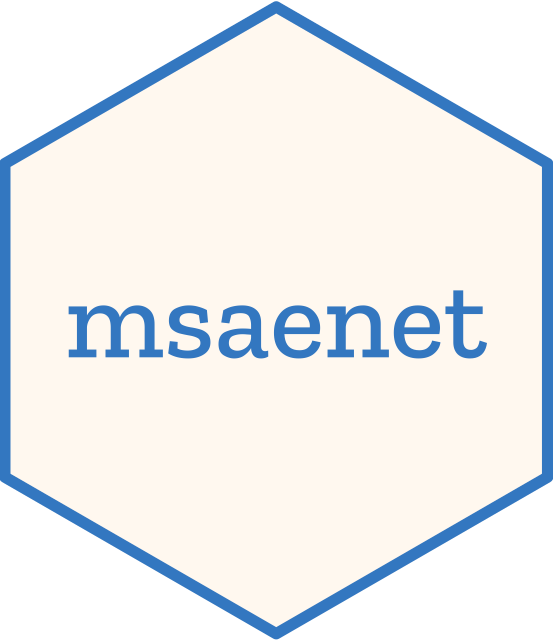
Package index
-
msaenet.sim.gaussian()
- Generate Simulation Data for Benchmarking Sparse Regressions (Gaussian Response)
-
msaenet.sim.binomial()
- Generate Simulation Data for Benchmarking Sparse Regressions (Binomial Response)
-
msaenet.sim.poisson()
- Generate Simulation Data for Benchmarking Sparse Regressions (Poisson Response)
-
msaenet.sim.cox()
- Generate Simulation Data for Benchmarking Sparse Regressions (Cox Model)
Model fitting
Fit adaptive and multi-step estimation models based on elastic-net, MCP-net, or SCAD-net penalties.
-
aenet()
- Adaptive Elastic-Net
-
msaenet()
- Multi-Step Adaptive Elastic-Net
-
amnet()
- Adaptive MCP-Net
-
msamnet()
- Multi-Step Adaptive MCP-Net
-
asnet()
- Adaptive SCAD-Net
-
msasnet()
- Multi-Step Adaptive SCAD-Net
-
msaenet-package
- msaenet: Multi-Step Adaptive Estimation Methods for Sparse Regressions
-
plot(<msaenet>)
- Plot msaenet Model Objects
-
predict(<msaenet>)
- Make Predictions from an msaenet Model
-
print(<msaenet>)
- Print msaenet Model Information
-
msaenet.nzv()
- Get Indices of Non-Zero Variables
-
msaenet.nzv.all()
- Get Indices of Non-Zero Variables in All Steps
-
msaenet.tp()
- Get the Number of True Positive Selections
-
msaenet.fp()
- Get the Number of False Positive Selections
-
msaenet.fn()
- Get the Number of False Negative Selections
-
coef(<msaenet>)
- Extract Model Coefficients
-
msaenet.rmse()
- Root Mean Squared Error (RMSE)
-
msaenet.mse()
- Mean Squared Error (MSE)
-
msaenet.mae()
- Mean Absolute Error (MAE)
-
msaenet.rmsle()
- Root Mean Squared Logarithmic Error (RMSLE)