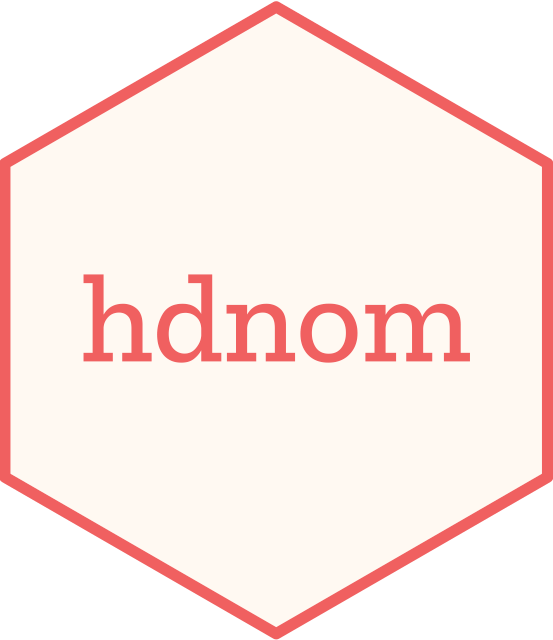
Model selection for high-dimensional Cox models with fused lasso penalty
Source:R/1_1_model.R
fit_flasso.Rd
Automatic model selection for high-dimensional Cox models with fused lasso penalty, evaluated by cross-validated likelihood.
Arguments
- x
Data matrix.
- y
Response matrix made by
Surv
.- nfolds
Fold numbers of cross-validation.
- lambda1
Vector of lambda1 candidates. Default is
0.001, 0.05, 0.5, 1, 5
.- lambda2
Vector of lambda2 candidates. Default is
0.001, 0.01, 0.5
.- maxiter
The maximum number of iterations allowed. Default is
25
.- epsilon
The convergence criterion. Default is
1e-3
.- seed
A random seed for cross-validation fold division.
- trace
Output the cross-validation parameter tuning progress or not. Default is
FALSE
.- parallel
Logical. Enable parallel parameter tuning or not, default is
FALSE
. To enable parallel tuning, load thedoParallel
package and runregisterDoParallel()
with the number of CPU cores before calling this function.- ...
Note
The cross-validation procedure used in this function is the
approximated cross-validation provided by the penalized
package. Be careful dealing with the results since they might be more
optimistic than a traditional CV procedure. This cross-validation
method is more suitable for datasets with larger number of observations,
and a higher number of cross-validation folds.
Examples
data("smart")
x <- as.matrix(smart[, -c(1, 2)])[1:120, ]
time <- smart$TEVENT[1:120]
event <- smart$EVENT[1:120]
y <- survival::Surv(time, event)
fit <- fit_flasso(
x, y,
lambda1 = c(1, 10), lambda2 = c(0.01),
nfolds = 3, seed = 11
)
#> 123123
nom <- as_nomogram(
fit, x, time, event,
pred.at = 365 * 2,
funlabel = "2-Year Overall Survival Probability"
)
plot(nom)