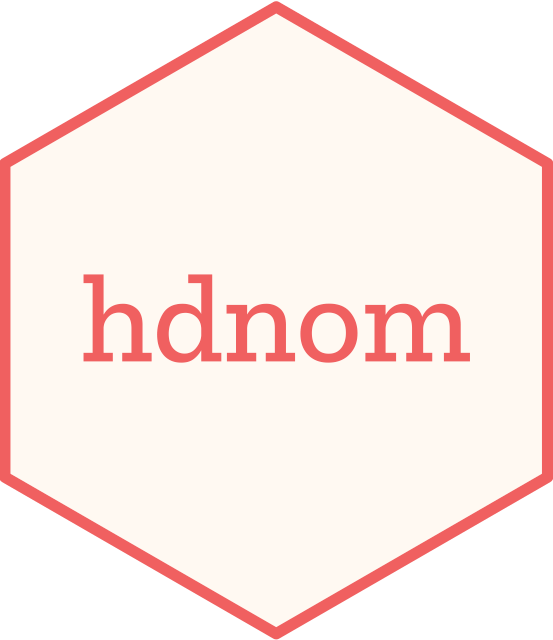
Compare high-dimensional Cox models by model validation
Source:R/5_1_compare_by_validate.R
compare_by_validate.Rd
Compare high-dimensional Cox models by model validation
Usage
compare_by_validate(
x,
time,
event,
model.type = c("lasso", "alasso", "flasso", "enet", "aenet", "mcp", "mnet", "scad",
"snet"),
method = c("bootstrap", "cv", "repeated.cv"),
boot.times = NULL,
nfolds = NULL,
rep.times = NULL,
tauc.type = c("CD", "SZ", "UNO"),
tauc.time,
seed = 1001,
trace = TRUE
)
Arguments
- x
Matrix of training data used for fitting the model; on which to run the validation.
- time
Survival time. Must be of the same length with the number of rows as
x
.- event
Status indicator, normally 0 = alive, 1 = dead. Must be of the same length with the number of rows as
x
.- model.type
Model types to compare. Could be at least two of
"lasso"
,"alasso"
,"flasso"
,"enet"
,"aenet"
,"mcp"
,"mnet"
,"scad"
, or"snet"
.- method
Validation method. Could be
"bootstrap"
,"cv"
, or"repeated.cv"
.- boot.times
Number of repetitions for bootstrap.
- nfolds
Number of folds for cross-validation and repeated cross-validation.
- rep.times
Number of repeated times for repeated cross-validation.
- tauc.type
Type of time-dependent AUC. Including
"CD"
proposed by Chambless and Diao (2006).,"SZ"
proposed by Song and Zhou (2008).,"UNO"
proposed by Uno et al. (2007).- tauc.time
Numeric vector. Time points at which to evaluate the time-dependent AUC.
- seed
A random seed for cross-validation fold division.
- trace
Logical. Output the validation progress or not. Default is
TRUE
.
References
Chambless, L. E. and G. Diao (2006). Estimation of time-dependent area under the ROC curve for long-term risk prediction. Statistics in Medicine 25, 3474–3486.
Song, X. and X.-H. Zhou (2008). A semiparametric approach for the covariate specific ROC curve with survival outcome. Statistica Sinica 18, 947–965.
Uno, H., T. Cai, L. Tian, and L. J. Wei (2007). Evaluating prediction rules for t-year survivors with censored regression models. Journal of the American Statistical Association 102, 527–537.
Examples
data(smart)
x <- as.matrix(smart[, -c(1, 2)])[1:1000, ]
time <- smart$TEVENT[1:1000]
event <- smart$EVENT[1:1000]
# Compare lasso and adaptive lasso by 5-fold cross-validation
cmp.val.cv <- compare_by_validate(
x, time, event,
model.type = c("lasso", "alasso"),
method = "cv", nfolds = 5, tauc.type = "UNO",
tauc.time = seq(0.25, 2, 0.25) * 365, seed = 1001
)
#> Starting model 1 : lasso
#> Start fold 1
#> Start fold 2
#> Start fold 3
#> Start fold 4
#> Start fold 5
#> Starting model 2 : alasso
#> Start fold 1
#> Start fold 2
#> Start fold 3
#> Start fold 4
#> Start fold 5
print(cmp.val.cv)
#> High-Dimensional Cox Model Validation Object
#> Random seed: 1001
#> Validation method: k-fold cross-validation
#> Cross-validation folds: 5
#> Model type: lasso
#> glmnet model alpha: 1
#> glmnet model lambda: 0.03893173
#> glmnet model penalty factor: not specified
#> Time-dependent AUC type: UNO
#> Evaluation time points for tAUC: 91.25 182.5 273.75 365 456.25 547.5 638.75 730
#>
#> High-Dimensional Cox Model Validation Object
#> Random seed: 1001
#> Validation method: k-fold cross-validation
#> Cross-validation folds: 5
#> Model type: alasso
#> glmnet model alpha: 1
#> glmnet model lambda: 3.330828
#> glmnet model penalty factor: specified
#> Time-dependent AUC type: UNO
#> Evaluation time points for tAUC: 91.25 182.5 273.75 365 456.25 547.5 638.75 730
#>
summary(cmp.val.cv)
#> Model type: lasso
#> 91.25 182.5 273.75 365 456.25 547.5 638.75
#> Mean 0.5514232 0.5750925 0.5908706 0.6486018 0.6315953 0.6615158 0.6475695
#> Min 0.1919192 0.3720636 0.4318947 0.5247777 0.4999633 0.5416780 0.5432238
#> 0.25 Qt. 0.4054983 0.4326005 0.5056009 0.5330110 0.5330110 0.5819999 0.5859288
#> Median 0.6362098 0.5369578 0.5155505 0.5450753 0.5461533 0.5949445 0.6091759
#> 0.75 Qt. 0.6742424 0.7018313 0.6757791 0.8073438 0.7434037 0.7520246 0.6958370
#> Max 0.8492462 0.8320093 0.8255278 0.8328010 0.8354450 0.8369321 0.8036820
#> 730
#> Mean 0.6292095
#> Min 0.5569337
#> 0.25 Qt. 0.5915718
#> Median 0.6105764
#> 0.75 Qt. 0.6746757
#> Max 0.7122901
#> 91.25 182.5 273.75 365 456.25 547.5 638.75
#> Mean 0.5514232 0.5750925 0.5908706 0.6486018 0.6315953 0.6615158 0.6475695
#> Min 0.1919192 0.3720636 0.4318947 0.5247777 0.4999633 0.5416780 0.5432238
#> 0.25 Qt. 0.4054983 0.4326005 0.5056009 0.5330110 0.5330110 0.5819999 0.5859288
#> Median 0.6362098 0.5369578 0.5155505 0.5450753 0.5461533 0.5949445 0.6091759
#> 0.75 Qt. 0.6742424 0.7018313 0.6757791 0.8073438 0.7434037 0.7520246 0.6958370
#> Max 0.8492462 0.8320093 0.8255278 0.8328010 0.8354450 0.8369321 0.8036820
#> 730
#> Mean 0.6292095
#> Min 0.5569337
#> 0.25 Qt. 0.5915718
#> Median 0.6105764
#> 0.75 Qt. 0.6746757
#> Max 0.7122901
#>
#> Model type: alasso
#> 91.25 182.5 273.75 365 456.25 547.5 638.75
#> Mean 0.5438734 0.6253119 0.6246102 0.6566693 0.6505558 0.6767926 0.6664697
#> Min 0.1161616 0.4108091 0.5219790 0.5361901 0.5334362 0.5378952 0.5394197
#> 0.25 Qt. 0.3891753 0.5148460 0.5289428 0.5577034 0.5361901 0.6129380 0.6162614
#> Median 0.7121212 0.5690552 0.5697782 0.5913443 0.6003684 0.6309677 0.6296160
#> 0.75 Qt. 0.7286432 0.8005930 0.6859652 0.7864692 0.7680248 0.7872057 0.7359087
#> Max 0.7732657 0.8312563 0.8163858 0.8116394 0.8147596 0.8149562 0.8111427
#> 730
#> Mean 0.6646070
#> Min 0.5620190
#> 0.25 Qt. 0.6241618
#> Median 0.6301660
#> 0.75 Qt. 0.7335408
#> Max 0.7731476
#> 91.25 182.5 273.75 365 456.25 547.5 638.75
#> Mean 0.5438734 0.6253119 0.6246102 0.6566693 0.6505558 0.6767926 0.6664697
#> Min 0.1161616 0.4108091 0.5219790 0.5361901 0.5334362 0.5378952 0.5394197
#> 0.25 Qt. 0.3891753 0.5148460 0.5289428 0.5577034 0.5361901 0.6129380 0.6162614
#> Median 0.7121212 0.5690552 0.5697782 0.5913443 0.6003684 0.6309677 0.6296160
#> 0.75 Qt. 0.7286432 0.8005930 0.6859652 0.7864692 0.7680248 0.7872057 0.7359087
#> Max 0.7732657 0.8312563 0.8163858 0.8116394 0.8147596 0.8149562 0.8111427
#> 730
#> Mean 0.6646070
#> Min 0.5620190
#> 0.25 Qt. 0.6241618
#> Median 0.6301660
#> 0.75 Qt. 0.7335408
#> Max 0.7731476
#>
plot(cmp.val.cv)
#> 91.25 182.5 273.75 365 456.25 547.5 638.75
#> Mean 0.5514232 0.5750925 0.5908706 0.6486018 0.6315953 0.6615158 0.6475695
#> Min 0.1919192 0.3720636 0.4318947 0.5247777 0.4999633 0.5416780 0.5432238
#> 0.25 Qt. 0.4054983 0.4326005 0.5056009 0.5330110 0.5330110 0.5819999 0.5859288
#> Median 0.6362098 0.5369578 0.5155505 0.5450753 0.5461533 0.5949445 0.6091759
#> 0.75 Qt. 0.6742424 0.7018313 0.6757791 0.8073438 0.7434037 0.7520246 0.6958370
#> Max 0.8492462 0.8320093 0.8255278 0.8328010 0.8354450 0.8369321 0.8036820
#> 730
#> Mean 0.6292095
#> Min 0.5569337
#> 0.25 Qt. 0.5915718
#> Median 0.6105764
#> 0.75 Qt. 0.6746757
#> Max 0.7122901
#> 91.25 182.5 273.75 365 456.25 547.5 638.75
#> Mean 0.5438734 0.6253119 0.6246102 0.6566693 0.6505558 0.6767926 0.6664697
#> Min 0.1161616 0.4108091 0.5219790 0.5361901 0.5334362 0.5378952 0.5394197
#> 0.25 Qt. 0.3891753 0.5148460 0.5289428 0.5577034 0.5361901 0.6129380 0.6162614
#> Median 0.7121212 0.5690552 0.5697782 0.5913443 0.6003684 0.6309677 0.6296160
#> 0.75 Qt. 0.7286432 0.8005930 0.6859652 0.7864692 0.7680248 0.7872057 0.7359087
#> Max 0.7732657 0.8312563 0.8163858 0.8116394 0.8147596 0.8149562 0.8111427
#> 730
#> Mean 0.6646070
#> Min 0.5620190
#> 0.25 Qt. 0.6241618
#> Median 0.6301660
#> 0.75 Qt. 0.7335408
#> Max 0.7731476
plot(cmp.val.cv, interval = TRUE)
#> 91.25 182.5 273.75 365 456.25 547.5 638.75
#> Mean 0.5514232 0.5750925 0.5908706 0.6486018 0.6315953 0.6615158 0.6475695
#> Min 0.1919192 0.3720636 0.4318947 0.5247777 0.4999633 0.5416780 0.5432238
#> 0.25 Qt. 0.4054983 0.4326005 0.5056009 0.5330110 0.5330110 0.5819999 0.5859288
#> Median 0.6362098 0.5369578 0.5155505 0.5450753 0.5461533 0.5949445 0.6091759
#> 0.75 Qt. 0.6742424 0.7018313 0.6757791 0.8073438 0.7434037 0.7520246 0.6958370
#> Max 0.8492462 0.8320093 0.8255278 0.8328010 0.8354450 0.8369321 0.8036820
#> 730
#> Mean 0.6292095
#> Min 0.5569337
#> 0.25 Qt. 0.5915718
#> Median 0.6105764
#> 0.75 Qt. 0.6746757
#> Max 0.7122901
#> 91.25 182.5 273.75 365 456.25 547.5 638.75
#> Mean 0.5438734 0.6253119 0.6246102 0.6566693 0.6505558 0.6767926 0.6664697
#> Min 0.1161616 0.4108091 0.5219790 0.5361901 0.5334362 0.5378952 0.5394197
#> 0.25 Qt. 0.3891753 0.5148460 0.5289428 0.5577034 0.5361901 0.6129380 0.6162614
#> Median 0.7121212 0.5690552 0.5697782 0.5913443 0.6003684 0.6309677 0.6296160
#> 0.75 Qt. 0.7286432 0.8005930 0.6859652 0.7864692 0.7680248 0.7872057 0.7359087
#> Max 0.7732657 0.8312563 0.8163858 0.8116394 0.8147596 0.8149562 0.8111427
#> 730
#> Mean 0.6646070
#> Min 0.5620190
#> 0.25 Qt. 0.6241618
#> Median 0.6301660
#> 0.75 Qt. 0.7335408
#> Max 0.7731476